We’ve reviewed countless data scientist resumes and have made a concerted effort to distill what works and what doesn’t about each of them.
Our number one tip to create an effective data science resume is to quantify your impact on the business! These 20 data scientist resume samples below and our data scientist cover letter templates can help you build a great job application in 2024, no matter your career stage.
Whether you’re looking for your first job as an entry-level data scientist or are a veteran with 10+ years of expertise, you’ll find plenty of tools to build your perfect resume, like our new Word resume examples or free Google Docs resume templates.
Why this resume works
- We can’t stress it enough. Quantify your impact!
- You need to write your resume in a way that shows the employer that you’ve materially impacted the companies you’ve worked for.
- This means you should quantify your value in terms of business impact, not model performance. Model performance metrics without context really don’t convey much.
- The numbers on your data scientist resume can be rough estimates.
- They’re a way to quickly display your achievements and convince the employer that you’ll bring that same kind of energy to their team or company.
Why this resume works
- The recruiter is hooked by your beautiful resume format, and even without much experience, you’ve quantified your successes so well you’ve left them open-mouthed. But you’re still not the well-rounded candidate they want, so there goes your dream role.
- For a splendid data science student resume, demonstrate a diverse skill set, prioritizing in-demand options (think Python, Jupyter Notebook, Pandas, Excel, SQL Server, etc.). Soft skills, ranging from teamwork and leadership to problem-solving, creativity, and adaptability are a welcome addition to your piece.
Why this resume works
- Considering adding projects to your entry-level data scientist resume in lieu of enough work experience?
- Great idea! Just be sure to discuss your projects in terms of their quantitative impact.
- You can demo the punch of a project by framing a question and then answering that question with data.
- Again, your results should be consistently expressed in numbers. Even if the result is as silly as saving 12 minutes per movie, it recognizes the importance of measuring impact.
- Top off your piece with a resume objective only if you’re willing to customize it to the job to which you’re applying.
- Customizing looks like: mentioning the target business by name and including relevant keywords from the job description.
Why this resume works
- Some people have few jobs in their experience sections, and yours might have next to none if you’re just breaking into your field. But don’t worry! You can make any internship or noteworthy project glow by paying extra attention to your skills section.
- When you have little to no professional background, the skills you list on your resume matter more than ever. And your abilities aren’t just selling points—they’re also a springboard for you to demonstrate your willingness to learn.
- Your “beginner” resume is also the perfect place to include a resume objective statement that shows your ambition.
- While writing your associate data scientist resume objective, immediately dive into any education or internship highlights with notable companies like Northrop Grumman. Then, sprinkle in some personality that shows your enthusiasm for new knowledge—drive and inquisitiveness are highly desirable traits in new professionals.
Why this resume works
- Remember, all experienced professionals have unique traits or skills that make them successful in their field, and data scientists are no different.
- Skip mentioning basic tasks like extracting information from your experienced data scientist resume. Rather, use phrases like “lowered maintenance costs” or “improved sentiment analysis accuracy” to showcase how you provide value to businesses and win for any prospective employer.
Why this resume works
- Your senior data scientist resume can really wow when you show a clear career progression from data analyst to data scientist to senior data scientist.
- That said, if you’ve got at least four years of experience under your belt, it’s fine for your work experience to account for about 70 percent of the page.
- Should you boast a whopping 10+ years of experience, you can opt to fill some of that remaining white space with a resume summary.
- A worthwhile summary should give a quick snapshot of your career highlights in two to three power-packed sentences and include the target company by name.
Why this resume works
- Companies don’t expect their interns to have extensive industry experience, so it’s not imperative to fully align your resume with your target role. But if you have relevant skills that you gained outside of your work experience, a career objective is an excellent place to highlight them.
- Call attention to your expertise in computer science by listing your proficiency in advanced programs like Keras on your data scientist intern resume.
Why this resume works
- A former Google recruiter once said, “A resume is showcasing you in a 10-to-60-second format on paper.” Creating a strictly text-based Google data scientist resume that doesn’t convey any achievements is only going to turn off would-be employers.
- So, to get adequate space to tell your story, use our elegant template and include subtle colors that are cool and yet don’t distract readers. Remember to use appropriate spacing and highlight your best achievements to keep hiring managers engaged with your career’s story.
Why this resume works
- If you don’t have any specialization courses under your belt, then it’s time to whip out quantified bullet points from your past roles.
- Whether it’s geospatial analysis, real-time data monitoring, or even creating standard visuals, make sure to quantify the impact of each and clearly state the benefit these tasks brought to the company to strengthen your data visualization resume.
Why this resume works
- Know what’s better than having one education qualification on a healthcare data scientist resume?
- Having two qualifications! Now’s the time to show all the degrees you’ve got! The best-case scenario is to have two degrees where one caters to the healthcare field while the other highlights your expertise in data science!
Why this resume works
- What sets your Amazon data science resume apart from the rest in the stack? Well, several things should but it’s the career objective that represents your first line of “attack”.
- Let that statement capture your aspirations and what you desire to bring to your new employer. Hiring managers are eager to see your passionate side and value to the team.
Why this resume works
- What’s your track record in being a project lead and ensuring that all goals are met within stipulated timelines? Because potential employers want someone with a background of success after success.
- Mentioning achievements such as improving project outcomes and reduction in process duration in your Python data scientist resume is a great way to leverage your experience honed over years of hard work.
- Then, by writing a great cover letter, you give yourself room to expound on exactly how you reduced process duration as a Python data scientist.
Why this resume works
- Even if you already have ample experience in your field, you can give your data scientist machine learning resume a competitive edge by bringing your higher education to light. Create space to showcase your advanced degree in a relevant subject like statistics to further stand out.
Why this resume works
- When seeking a data science manager role, it makes sense that your work experience focuses on leadership and project ownership.
- Again, the results of your work should be stated clearly in terms of tangible impact (are you sensing a theme?).
- While content is king, appearance is queen.
- Using a two-column layout for your data science manager resume allows more information to fit on a single page. Even with nine-plus years of experience, keeping your resume to one page is ideal.
- Fretting these details? Our resume templates for 2024 may suit your specific needs; additionally, we’ve got fresh and free Google Docs resume templates that can make your resume-creation blues go away!
Why this resume works
- When you’re trying to figure out what to put on your resume for a more specialized role like an NLP data scientist, it’s important you showcase your proficiency in operationalizing models to have a big impact on the business.
- Remember, the goal of any data scientist (including NLP experts) is to deploy models to make positive impacts on product and user experience.
- Don’t focus on the technical aspects of the models you’ve built on your NLP data scientist resume (you’ll talk more about that in your interviews). Instead, take a step back and talk about the broad impact you’ve had in your previous roles.
Why this resume works
- Entry-level metadata scientist roles can be difficult to land. However, your metadata scientist resume can be an excellent way to display large-scale data-manipulation skills required by the industry.
- Prove your experience in programming, testing, modeling, and data visualization through well-designed projects that solve real problems through code.
- The key isn’t to reinvent the wheel but to create something dynamic and unique that isn’t easily replicated with a few Google searches and a video tutorial.
- Though related work experience is preferred when you make a resume, entry-level candidates often share a common denominator: a light or nonexistent job history in the field.
- Solve this problem with projects. If you’ve worked on excellent projects that used and showcased the necessary skills required for the job, list them and watch your resume bloom with confidence!
Why this resume works
- Talk about a great career summary! If you decide to include a resume summary in your educational data scientist resume, dazzle the reader with solid achievements that speak to their own relevance within the field.
- Think “well-rounded” as you write; you might include an exciting publication related to the job role, quickly outline your relevant experience or abilities, and conclude with how and why you’ll better the company through your new role.
- Use your resume to not just list what you’ve done in previous jobs, but articulate how you bettered your previous work environments.
- Skills and certifications add credibility, but potential employers also want to know about your impact.
- If you performed evaluations, what improvements did you make afterward? If you integrated machine learning, what optimizations did you use it for?
Why this resume works
- The first thing recruiters will notice when reading your resume is whether the fundamental qualifications to perform the job adequately are there.
- Your data scientist, analytics resume should target the list of requirements that companies in your state commonly request.
- For example, 18 out of 20 job descriptions for data science, analytics in the state of California list Python, SQL, R, Tableau, and Hadoop (in that order) as required skills.
- After you add job-market-specific data, our free resume checker can assess your resume for other key elements like spelling, grammar, and active language.
Why this resume works
- Your work experience is the most crucial component of your data science consultant resume.
- To best represent your capabilities, use metrics to talk about your accomplishments.
Why this resume works
- As a data science director, you will have a lot of experience under your belt. Remember that it’s not necessary to add every single thing you’ve ever done to your resume.
- For an effective data science director resume, use a clean and simple resume template and format your work experience in reverse-chronological order. Doing so will put your most recent and relevant accomplishments at the top, making it the first thing a recruiter will look at.
Related resume guides
Writing Your Data Scientist Resume
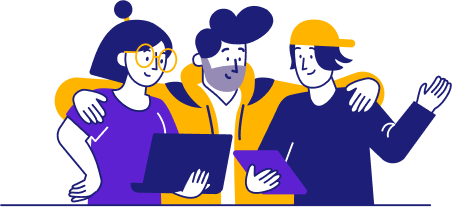
Recruiters only spend an average of seven-plus seconds reviewing your resume, so it’s vitally important that you catch their attention in that time. Our guide for 2024 takes you section by section through your resume to ensure you get that first interview.
You can successfully choose a winning resume format in 2024 that will snag an employer’s attention.
Short on time? Here are the quick-hit summaries of each section you can apply to your resume:
- Projects & Work Experience
- Whether for a company or yourself, what you’ve worked on should be the focus of your resume. Always try to include a measurable impact of your work.
- Summary/ Headline/ Objective
- Make this the job title you’re looking for (e.g., “data scientist”), and don’t worry about a summary unless you’re making a career change.
- Skills
- Only include technical skills that you’d be comfortable having to code with/in during an interview. Avoid a laundry list of different skills.
- Education
- Include relevant courses if you’re looking for an entry-level role. Otherwise, make your work the focus of your resume. If you went to a boot camp, list it here.
- Contact Info
- Double-check everything. This is not the place you want to make a mistake. You don’t need to put your exact address. City, state, and zip are fine.
- General Formatting Tips
- Try to keep it to one page. Keep your bullets brief. Triple-check your grammar and spelling, and then have someone else read it.
- Customization for Each Job
- Read the data scientist job description. See if any projects you’ve worked on come to mind while reading it. Incorporate those specific projects into your resume.

Your data science projects and work experience
Let’s jump right into the good stuff and talk about the most important part of your resume: your work experience and projects. This is it. This is the grand finale. This is where the person reviewing your resume decides whether or not you’ll get an interview.
When talking about your previous work (whether that’s for another employer or on a side project), your goal is to convince the person reviewing your resume that you’ll provide value to their company. This is not the place to be humble. We want to see that “I’m wearing my favorite outfit” level of confidence.
The template for successfully talking about your experience as a data scientist is:
- Clearly state the goal of the project
- Demonstrate what you did
- You can mention the programming languages you used, the libraries, modeling techniques, data sources, etc.
- State the quantitative results of your project
You’re a data scientist, so highlight your value by demonstrating the quantitative impact of your work. These can be estimates. For example, did you automate a report? Roughly how many hours of manual work did you save each month? Here are some ideas for how you can quantitatively talk about your projects:
Ways to define the impact of your data science work
- Increased revenue
- Example: You developed a pricing algorithm that resulted in a $200k lift in annual revenue.
- Improved retention or conversion rate
- Example: You built a model to predict who would cancel their subscription and introduced an intervention to improve monthly retention from 90% to 93%.
- Increased growth
- Example: You built a marketing attribution model that helped the company focus on marketing channels that were working, resulting in 2,100 more users.
- Improved engagement rate
- Example: You ran an experiment across different product features, which resulted in a 25% increase in engagement rate.
- Saved labor
- Example: As a side project, you built a movie recommendation engine that now saves you 26 minutes each time you need to decide which movie to watch.
- Lift in consumer satisfaction
- Example: Since you built a customer segmentation model to determine how to communicate with different customer types, customer satisfaction is up 17%.
Numbers draw attention, are convincing, and make your resume more readable. Which of these two ways to describe reporting is more compelling?
- Used Python, SQL, and Tableau to conduct daily reporting for the business
- Using Python, SQL, and Tableau, combined 11 data sources into a comprehensive, real-time report that saved 10 hours of work weekly
If nothing else, please take this away from this guide: state the results of your projects on your resume in numbers.

Trade-offs between projects and work experience
Simply put, the more work experience you have, the less space “projects” should take up as a section on your resume. In the sample resumes above, you’ll notice that only the more entry-level data scientist resumes have a section for projects.
The senior-level resumes focus on projects in the context of experience within companies. Real estate is precious on a one-page resume, so you’ll want to focus on the bullets that most clearly demonstrate how you’re a great fit for the job. Companies want to hire data scientists who have demonstrated success at other companies.

Entry-level data science projects for resume
Junior data scientists should include projects on their resumes. Try starting with a resume outline, where you can brain dump anything and everything about your projects; then, you can distill the best of it into your final resume. Can you share the Github link? Do you have a link to a write-up you did about your project?
The more initiative you can show for entry-level data science projects, the better. Do you have any questions to which you’ve always wanted the answer? You can probably think of some clever ways to get data around that question and come up with a reasonable answer. For example, our co-founder wanted to know which data science job boards were best, so he pulled together some data, laid out his assumptions and methodology, and made his conclusions.
No matter what projects you include on your resume, be sure to clearly state the question you were answering, the tools and technologies you used, the data you used to answer the question, and the quantitative outcome of the project. Succinctly stating conclusions and recommendations from your analysis is a highly sought-after skill by employers in data science.

The data scientist summary
Since you have limited space on your resume, you should only include a resume objective if you take the time to customize it for each role to which you apply.
You may want to include a resume summary or objective when you’re making a big career change. If you do include one, make sure to keep it specific about your goal and experience. This is valuable space you’re going to be using on this statement, so take the time to personalize it to each job.
Include the title of the job you’re looking for under your name. This should be aspirational. So if you’re a data analyst looking to apply for data scientist jobs, you would put “data scientist” under your name as the headline:
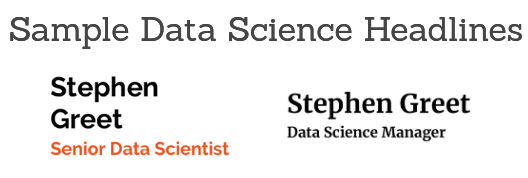

Skills that pay the bills
The most common mistake we see on data science resumes (that we used to make on our resumes) is what we call skill vomit. It’s a laundry list of skills in which no one person could have expertise. A quick rule of thumb: if the skills section takes up a third of the page, it takes too much space. This is a big red flag for hiring managers.
The reason people make such an exhaustive skills section is to get through the mythical data science resume keyword filters. If you’re changing your resume in small ways for each job you apply to (for example, put Python for jobs that mention Python and R for jobs that list R if you know both), you’ll have no problem with those keyword filters.
The rule of thumb that we recommend you use in determining whether to include a skill on your resume is this: if it’s on your resume, you should be comfortable coding with/in it during an interview.
So that means if you’ve read a few articles on Spark or adversarial learning, but you can’t use them in code, they should not be on your resume. If you only have a handful of tools under your toolbelt, but you can use them effectively to answer questions with data, you’ll be able to find jobs looking for that skill set.
We can assure you there are all kinds of data science jobs available. Our scraper that indexes jobs across thousands of company websites shows over 5,000+ full-time data science job openings in the US across all tenures and skill sets. And our scraper has a lot of room for improvement, so that’s significantly lower than the actual number.
There are tons of fish in the job market sea; you just need a fishing rod.

Entry-level vs. senior skills sections
Generally, the more senior you are, the shorter your skills section needs to be. If you’re a senior data scientist, you should talk about the major tools and languages you use but save specific modeling techniques for the “Work Experience” section. Show how you used particular models in the context of your work.
When you’re more junior, you likely haven’t had the chance to use all of the techniques you’re comfortable with within work or a project. That’s okay! It’s expected. But you still want to make it clear to a potential employer that you can use those methods or libraries.
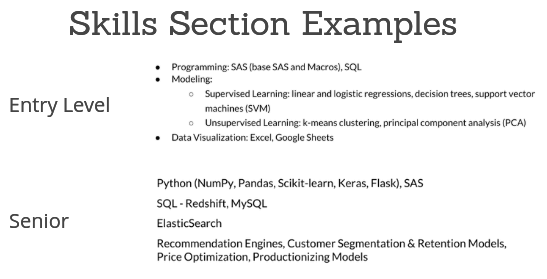

Education
Education is a lot like skills in that the more senior you are as a data scientist, the less space the education section should take up on your resume. When you’re looking for one of your first data science jobs, you might want to include courses relative to data science to demonstrate you have a strong foundation.
Classes in subjects like linear algebra, calculus, probability, and statistics and any programming classes are directly relevant to being a data scientist. If you’re looking for your first job out of college, you should include your GPA on your resume. When you have a few years of work experience, it’s not necessary to include it.
If you just finished (or are finishing) a data science boot camp, this is the place to list where you went. You can include the relevant lessons or classes you took. Be sure to have a few projects from your boot camp (especially if it was an original project) in your resume’s “Projects” section.
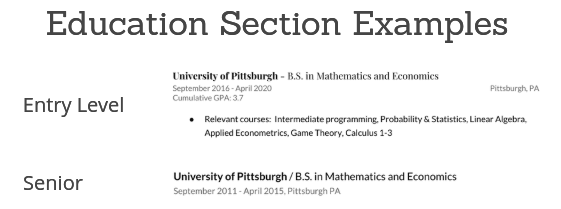

Contact information
The takeaway from this section is simple: this is not where you should make a mistake. Storytime! When our co-founder was first applying to jobs out of college, he realized about 20 applications in, he had spelled his name “Stepen” instead of “Stephen.” Don’t pull a Stepen.
Data suggests that when your email is wrong, your response rate from companies drops to zero percent. That’s just math. We’ve seen exactly four data science resumes where the email address on the resume was incorrect.
Make sure your email address is appropriate. While we don’t doubt the authenticity of your “[email protected]” email, maybe don’t use it when applying for jobs. To play it safe, stick to a combination of your name and numbers for your email.
This is the section you can include anything you want to show off for a data science role. Have a blog where you document the analysis you do for Dungeons & Dragons? Active on Github or an open-source project? Include a link to anything relevant to data that will help you stand out in your application.

General resume formatting tips
This section is just a list of one-off styling and formatting tips for your data science resume:
- Keep it brief. Bullets should be informative but should not drag on for paragraphs.
- Each bullet point in your resume should be a complete thought. You don’t have to have periods at the end of each bullet.
- Keep your tense consistent. If you’re referring to old projects in the past tense, do that for all old projects.
- Please, please don’t get your contact information wrong.
- Use Grammarly or similar to check the spelling and grammar in your resume. Then do that two more times. Finally, have someone else review it just for spelling and grammar.
- Don’t give the person reviewing your resume a silly reason to put it in the “No” pile. Check your resume carefully.

Customization for each application
You don’t have to go overboard with your resume customization. Here are the steps we recommend to customize it for each job:
- Ensure that for each language you have extensive experience in (Python and R, for example), you have separate resumes emphasizing specific projects in each language.
- So in this example, we’ll have one “Python” resume and one “R” resume depending on what the job is seeking.
- Read the data scientist job description. Do any specific projects you worked on come to mind as you read it? If so, include those projects as bullet points on your resume. Here are some sample questions to help you think of specific projects to list for different jobs:
- For example, if you have experience with attribution modeling and this is a marketing data science role, you should include that experience.
- Do you have experience with a certain library or modeling technique they mention?
- Do you have experience in the domain of the specific job?
- Do you have any relevant industry experience with the company?
Let’s walk through a specific example to highlight what we mean by including particular projects for different jobs. Let’s say that a senior data scientist is applying for the position below.
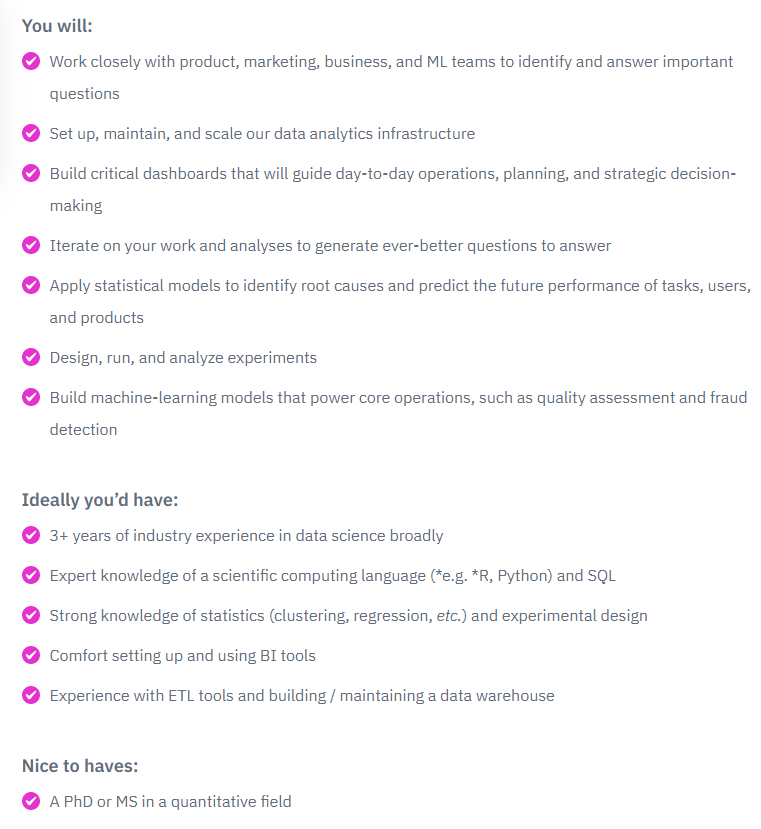
In the “Ideally, you’d have” section, they mention they want someone who has “Experience with ETL tools.” Let’s say that in reality, the candidate had a large role in building out data pipelines in his fictional role as a senior data scientist at EdTech Company.
So all we’d do is change that section of his experience at EdTech Company to talk about that project, as you see below:
Data science resume customization example
Original bullet on the resume: Worked closely with the product team to build a production recommendation engine in Python that improved the average length on the page for users and resulted in $325k in incremental annual revenue
Customized for the role: Built out our company’s ETL pipeline with Airflow, which scaled to handle millions of concurrent users with robust alerting/ monitoring

Customization for startups
For early-stage startups (anything less than 50 employees), one of the most important qualities they’re looking for in a hire is ownership. That means they want someone who can ask a question and come up with an answer with minimal instruction.
If you want to stand out to these companies, you should demonstrate ownership in the way you list projects on your resume. Include active words like “drove” or “built” instead of passive language like “worked on” or “collaborated on.” We know this seems nit-picky, but this matters to early-stage companies. Hiring managers at companies this size are strained for time and will use any signal to weed people out.

Concluding thoughts
There you have it—a compelling, easy-to-read data science resume built for 2024. Now you can celebrate by doing something as fun as writing a resume. Maybe your taxes? Or go to the dentist?
By building or updating your current resume, you took a huge step toward landing your next (or first) data science job. Now please, we beg you, check your grammar and spelling again and have someone else read your resume. Don’t let that be the reason you don’t get an interview.
Congrats! The first and hardest step is done. You have a data science resume! With great power comes great responsibility, so go and apply wisely.
Land your next job with our AI-powered, user-friendly tool.
Gut the guesswork in your job hunt. Upload your existing resume to check your score and make improvements. Build a resume with one of our eye-catching, recruiter-friendly templates.
• Work in real-time with immediate feedback and tips from our AI-powered experience.
• Leverage thousands of pre-written, job-specific bullet points.
• Edit your resume in-line like a Google Doc or let us walk you through each section at a time.
• Enjoy peace of mind with our money-back guarantee and 5-star customer support.